How often do AI Hallucinations happen?
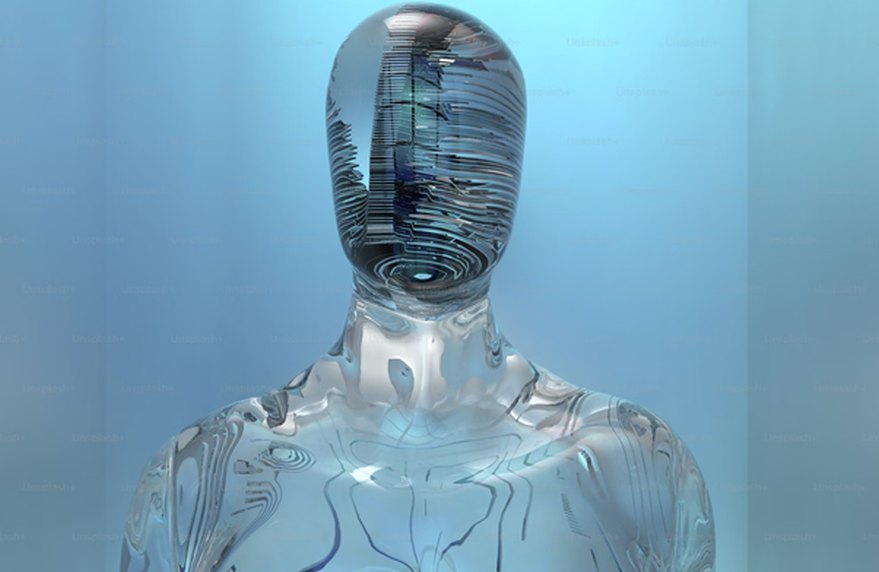
Artificial Intelligence (AI) has become an integral part of modern technology, running through a number of various sectors from healthcare to finance, and even creative industries. Despite its impressive capabilities, AI is not without its flaws.
As a copywriter, I find it hard to get my head around one significant issue: The occurrence of “hallucinations”. AI systems produce incorrect instructions or copies that I can’t understand. Understanding the frequency and nature of these ‘hallucinations’, and how to prevent or fix them, can be critical for optimizing AI performance and trustworthiness across websites and brands.
What is an AI hallucination?
AI hallucinations refer to instances when an AI system generates information that is not based on the input data or the knowledge it has been trained on. These hallucinations can manifest as factual inaccuracies, logical inconsistencies, or completely fabricated content which can be detrimental to brands and businesses, especially when Google algorithms come into play. They are particularly prevalent in generative models like OpenAI’s GPT series or image generation tools that defeat the point of creativity and those who work in these industries.
What causes AI hallucinations?
The frequency and sources of AI hallucinations can depend on multiple facets, such as;
Complexity
More complex models, while often more capable, are also more prone to hallucinations. The nature of how they are structured and their architectures can sometimes lead to unexpected outputs, especially in ambiguous or poorly defined contexts. So being clear and writing and searching can be a factor in how AI responds.
Data Quality
The quality of data has always been hard to get around, especially after new legislation and the protection of customers’ data changes. So training of data and how it’s handled significantly affects hallucination rates. Models trained on high-quality, well-curated data tend to hallucinate less frequently than those trained on noisy or biased datasets.
Tasks
Different tasks have varying susceptibility to hallucinations. For example, AI systems used in creative writing or image generation are more likely to hallucinate compared to those used in structured environments like chess when they can calculate the dynamics mathematically and not in a creative environment. A platform such as Aporia’s guardrails can be a great solution in ensuring that creatives can be protected against this and generate clients that value their work as creative individuals and support what they’re trying to achieve in the new space of the digital marketing world.
Users
Similarly, ambiguous or poorly phrased sentences from those who use AI can increase the likelihood of hallucinations. Clear, specific prompts generally result in more accurate responses.
Measuring the Frequency of AI Hallucinations
Measuring how often AI hallucinations happen can be challenging for the best of us, even as technology advances and it can depend on context and how it is used. However, studies and user reports provide some insights.
Academically, there’s a lot of research that comes with AI models from Chat GPT 3-4 and different webinars claiming AI hallucinations have always been around, whether that’s factual or nonsensical. As a result, just like media culture, the frequency of AI hallucinations can be more frequent than we think.
It’s been estimated that it can be as often as 15-20% in some contexts, which can largely impact the reputation of any business or brand.
Similarly to, user reports and benchmark tests can dramatically affect the outcome of AI and how it responds to user questions. Which can significantly impact how hallucinations manifest in software and technology.
How can we reduce AI hallucinations?
To reduce the occurrence of AI hallucinations, we can do a lot as humans to help.
- Improve data: Ensuring data can be up to date and free from errors can help to reduce mistakes and the level of hallucinations.
- Adding Guardrails: Guardrails could be a great way to safeguard the AI from a third-party tool and intercept and rephrase hallucinations in real time.
- Continue to refine AI: Ongoing refinement and rewriting of how AI software works can help to reprogram and improve this technology. This needs human feedback and writers to make sure the outputs are aligned more closely with what a human will suggest or expect to hear.
- Understanding prompts better: Educating how users can utilize AI and write better prompts, much like we did with Google and SEO can help systems to work more effectively.
- Analysis: Without data and analysis of what works and monitoring and assessing we can never assess or address flaws and then address or make improvements.
The Future of AI Hallucinations
As AI technology evolves, and the use of better prompts, guardrails, and data improvements, the frequency and impact of hallucinations are expected to diminish. Advances in model architectures, training methodologies, and data quality will likely lead to more reliable and accurate AI systems. Additionally, increased awareness and understanding of hallucinations among developers and users will contribute to better management and mitigation strategies.
In conclusion, while AI hallucinations are a notable challenge, their frequency varies depending on several factors, including model complexity, training data quality, and task type. By improving training data, refining models, enhancing user prompts, and implementing robust evaluation methods, the occurrence of hallucinations can be significantly reduced. As AI technology continues to advance, we can expect more reliable and trustworthy systems, enhancing the overall user experience and expanding the potential of AI applications.