Most AI startups are doomed to fail: Here’s why this venture capitalist thinks so
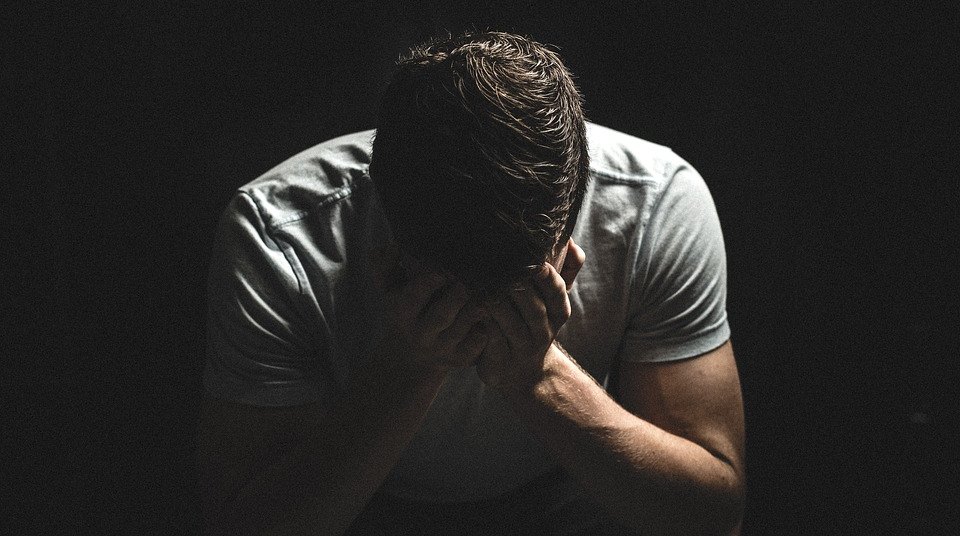
Since its launch on November 30, 2022, ChatGPT’s popularity has spurred the creation of numerous artificial intelligence (AI) startups. The past year witnessed a substantial influx of investments from both investors and venture capitalists into top generative AI startups. According to PitchBook data, funding for generative AI startups reached $21.4 billion by September 30 this year, a significant increase from the $5.1 billion invested in 2022.
The rise of these generative AI startups underscores the growing significance of AI-powered content generation in the current technology landscape. These startups contribute to the evolution and progress of AI, presenting exciting opportunities for businesses to explore and harness the potential of generative AI in their operations. However, the crucial question looms: how many of these startups will succeed, and how many will fail? Historical data suggests that 90 percent of all startups meet failure. By extension, does this imply that the majority of AI startups will face a similar fate?
James Wang, a General Partner at Creative Ventures and Co-Founder of Lioness, with prior experience at Bridgewater and Google X, holds a pessimistic view. In his piece titled “Most AI startups are doomed,” Wang asserts that a significant portion of today’s generative AI startups, particularly those emerging in the post-ChatGPT hype and explicitly labeling themselves as “AI startups,” are destined for failure.
Wang, a venture capitalist deeply involved in AI investments, argues that the surge of startups riding the ChatGPT hype and proudly flaunting their “AI” label are on a path to failure. While he isn’t inherently doubtful about AI, he contends that a significant portion of the current AI funding trend lacks substance from an investor’s perspective.
How AI Startups Are Using LLMs to Create AI Wrappers
Wang didn’t stop there. He also extends this argument to more intricate projects involving underlying technologies like large language models (LLMs) such as ChatGPT, Bard, and LlaMA. He questions the defensibility of these technologies, highlighting the process of gathering internet text, training it with significant computational resources, and using well-known, often open-source technologies. Wang argues that this lack of defensibility means any large internet company can replicate such models, citing statements from Alphabet/Google acknowledging this reality.
“These companies are also all doomed to either be perfectly ok businesses (but not startups, by Paul Graham’s classic definition) or die. Obviously, if you built it over a weekend, someone else can do the same. Now, let’s say you’re a coding genius. A veritable 10X programmer prodigy! It might take everyone else in the world several weekends… but it’s going to get built.”
The idea is explored that having the best version of an AI might not secure a sustainable advantage. Drawing parallels to having the fastest CPU in the 1990s, he suggests that maintaining a continuous advantage is challenging given the rapid evolution of AI technology. The competitive landscape, marked by open-source contributions and global research communities, makes it challenging for any single company to maintain a lasting edge.
Value Created Doesn’t Mean Value Captured
Wang also points out two potential areas where startups could find defensibility and value: substantial computational capabilities and real-world, proprietary data. However, he expresses reservations about the feasibility and desirability of a strategy based on accumulating more computational resources than major tech giants. On the other hand, he also sees promise in startups operating in domains where data cannot be easily sourced from the internet, such as healthcare, protein folding, or other areas requiring real-world experimentation.
Conclusion
The article concludes by underscoring that while societal value may be generated in the AI sector, it doesn’t guarantee that individual companies will capture that value. Wang highlights that some AI-related value may benefit existing industry incumbents, creating a scenario where societal gains surpass individual company profits. The article suggests that only a select group of AI startups—those effectively generating and capturing value, potentially displacing incumbents—will experience substantial returns and gain recognition in the tech landscape. He expresses concern that, in the current investment climate, indiscriminate funding of AI startups may lead to wasted resources.