Q-Learning: Advancing Towards AGI and Artificial Superintelligence (ASI) through Reinforcement Learning
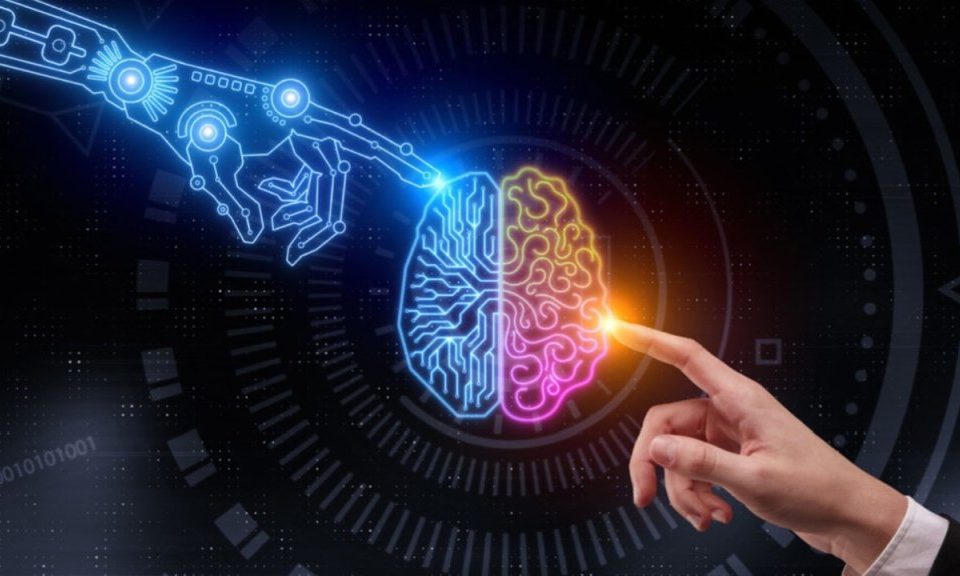
Earlier today, we covered the news about OpenAI reportedly making a secret artificial intelligence breakthrough dubbed Q*, or Q-Learning, as part of its journey towards achieving Artificial General Intelligence (AGI). The discovery was so significant that several researchers on the OpenAI team wrote a letter to the board of directors, expressing concerns that the discovery could pose a threat to humanity.
In addition, Reuters reported that the ‘super intelligence’ breakthrough was so big that it nearly destroyed the company and also played a role in the removal of Sam Altman from his position. While AGI has gained mainstream attention in recent months due to the popularity of ChatGPT, the concept of artificial superintelligence (ASI) is still relatively new. ASI is one of the three major types of artificial intelligence (AI) which includes artificial narrow intelligence (ANI), artificial general intelligence (AGI), and artificial super intelligence (ASI).
Artificial General Intelligence
Achieving Artificial General Intelligence (AGI) means reaching a point where the technology matches the cognitive abilities of a human mind. Given this similarity, understanding AGI might take a while, mainly because we’re still unraveling the complexities of the human brain. While we’re not there yet in terms of a full grasp, the idea behind AGI is that it could think at a human level, akin to Sonny, the robot in “I, Robot” starring Will Smith.
Artificial Superintelligence
Unlike ANI (simply AI) and AGI, artificial superintelligence (ASI) is like the ultimate version of AI, where a software system surpasses human intellectual abilities in various categories and fields. It’s crucial to note that ASI is still a hypothetical concept, meaning it doesn’t actually exist at this point.
This is distinct from regular artificial intelligence (AI), which focuses on simulating human intellectual capabilities through software. This includes tasks like learning from information, reasoning, and self-correction. Now, let’s dive into the main point of this story: What is Q-Learning, and why is it critical in the journey towards achieving Artificial General Intelligence (AGI) and Artificial Superintelligence (ASI)?
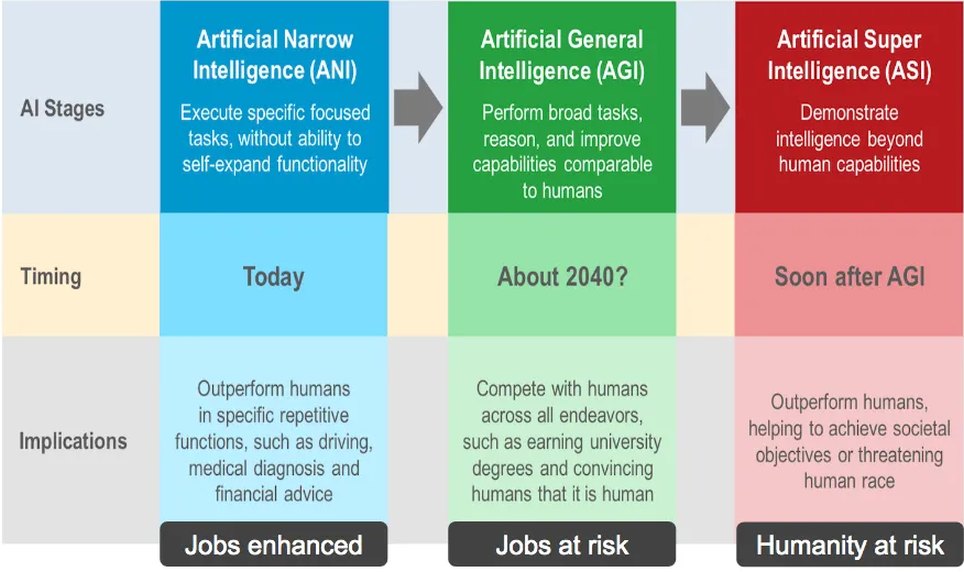
Types of AI: ANI, AGI, and ASI (Credit: Lawtomated)
What Is Q-Learning?
In the vast realm of artificial intelligence and machine learning, Q-Learning is a reinforcement learning algorithm that helps computers learn by trial and error, allowing them to make strategic decisions and maximize rewards. Think of Q-Learning as a clever way to teach computers how to play games. It works by giving the computer a set of rules and rewards and then letting it play the game over and over again. As the computer plays, it keeps track of which actions lead to the biggest rewards. Eventually, the computer learns to play the game well by choosing the actions that lead to the most rewards.
In the world of artificial intelligence and machine learning, Q-learning is a reinforcement learning algorithm that helps computers learn by trial and error and through experience. It enables computers to make smart decisions and aim for maximum rewards. You can think of Q-Learning as a nifty method for teaching computers how to excel in games.
Here’s how it works: The computer is given a set of rules and rewards, and then it dives into playing the game repeatedly. While playing, it keeps tabs on which actions result in the best rewards. Over time, it gets the hang of the game and becomes quite the expert, consistently picking actions that lead to the highest rewards. It’s like the computer learns the game’s tricks by figuring out which moves bring in the most prizes.
The Basics of Q-Learning
At its essence, Q-learning is akin to introducing a reward system to a computer, aiding it in deciphering the most effective strategies for playing a game. This process involves defining various actions that a computer can take in a given situation or state, such as moving left, right, up, or down in a video game. These actions and states are meticulously logged in what is commonly referred to as a Q-table.
The Q-table serves as the computer’s playground for learning, where it keeps tabs on the quality (Q-value) of each action in every state. Initially, it’s comparable to a blank canvas – the computer embarks on this journey without prior knowledge of which actions will lead to optimal results.
Exploration and Learning
The adventure commences with exploration. The computer takes a plunge into trying out different actions randomly, navigating the game environment, and recording the outcomes in the Q-table. Think of it as the computer playfully experimenting and gradually figuring out the lay of the land.
Learning from Rewards forms the core of Q-learning. Each time the computer takes an action, it earns a reward. The more effective the action, the greater the reward. The Q-table undergoes updates based on these rewards, symbolizing a learning process where the computer memorizes which actions result in favorable outcomes and refines its strategy accordingly.
Balancing Exploration and Exploitation
Continuing its gameplay, the computer encounters a delicate equilibrium between exploration and exploitation. Initially, it explores the game, grasping the consequences of different actions. As it acquires knowledge, it shifts gears towards exploitation – opting for actions that it recognizes will likely yield higher rewards.
Optimal Policy
With the passage of time and numerous iterations, the computer begins to decipher the most effective actions to take in each state to maximize its total rewards. This collection of optimal actions is known as the policy. Through Q-learning, the computer transforms from a novice to a strategic player, making informed decisions to attain the highest possible rewards.
Conclusion
Q-learning emerges as a robust tool that not only enables computers to partake in gaming but also equips them to adapt and thrive in diverse scenarios. Whether it involves navigating a video game or addressing real-world problems, Q-learning showcases the potential of machine learning to facilitate intelligent decisions through a process of exploration, learning from rewards, and refining strategies over time. In our ongoing exploration of artificial intelligence, Q-learning stands out as a captivating approach that seamlessly connects the realms of playing and learning in the digital landscape.
You can learn more about Q-Learning in the video below.