Semiconductor startup Syntiant raises $25 million Series B joined by Sunstone Venture Capital, Microsoft and Intel
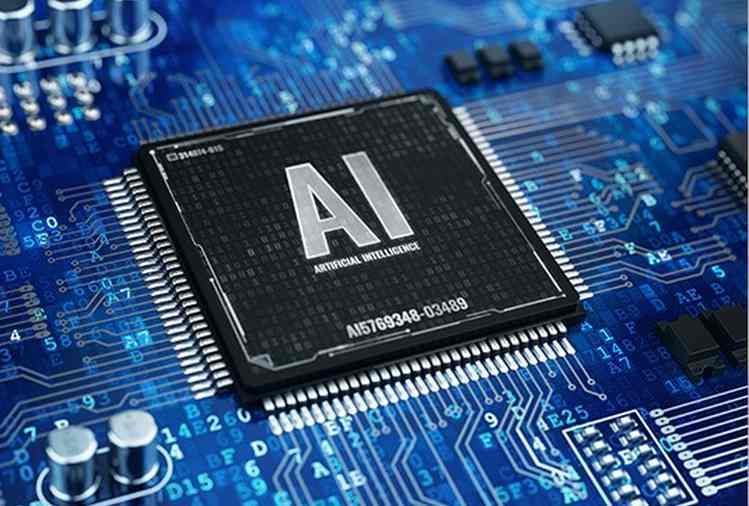
Syntiant Corp. (Syntiant), a new breed of semiconductor company that combines the best of deep learning and semiconductor design, to advance machine learning capacities in edge devices, has closed $25 million Series B to support its 2018 growth. The latest round is joined by Sunstone Venture Capital Fund, which also invested in Series A, alongside partner Seraph Group and other influential investors including Microsoft and Intel.
Syntiant is not your typical semiconductor company. Its semiconductors are used with artificial intelligence (AI) with key features such as: very low consumption, high performance and integration with neural networks of processors. Utilizing patented analog neural network techniques in flash memory, Syntiant’s Neural Decision Processors (NDP) completely eliminates data movement penalties by performing neural network computations in flash memory, enabling larger networks at significantly lower power. Its ultra-low power, high performance NDPs are ideal for always on applications in battery powered devices such as: smartphones, wearables, ear buds, remote controls, drones, security cameras, and sensors.
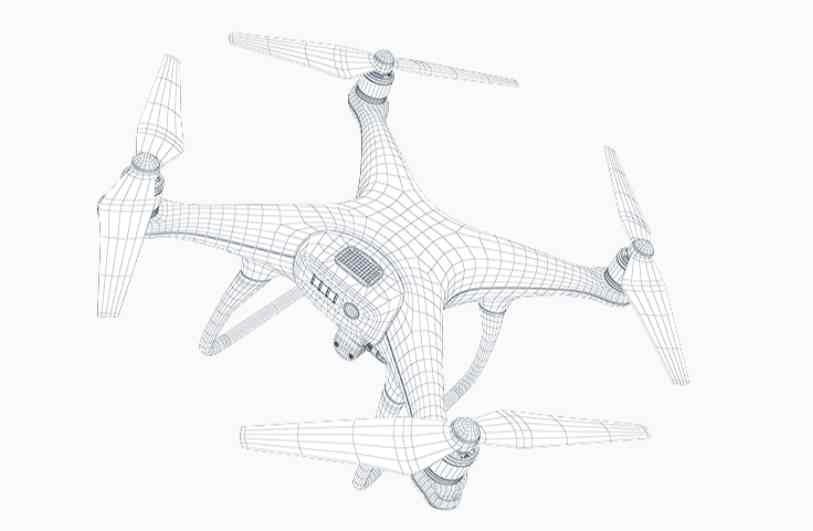
Syntiant’s NDP application in drone
Investors have also taken notice. Other giants of the world of technology have invested in this company including Amazon Alexa Fund, Applied Ventures, Intel Capital, Motorola Solutions Venture Capital and Robert Bosch Venture Capital. In conjunction with the funding, Syntiant announced that Samir Kumar, director of M12, will be joining its board.
Founded in 2017 by Jeremy Holleman, Kurt Busch, and Pieter Vorenkamp, Syntiant combines innovations in deep learning and semiconductor design to advance machine learning capacities in edge devices like smart phones, watches, speakers and more in IoT. The company’s analog neural networks expand the power of artificial intelligence to low-power semiconductors for battery-powered devices ideal for demanding always-on voice, sensor and image tasks. Syntiant breaks the chains of digital data path architectures with massively parallel neural networks ideally suited for high computational workloads required for real time applications. Its solutions are ideal for demanding always-on voice, sensor and image tasks.
Sunstone, a diversified capital management and investment firm based in Long Beach, California, participated in Syntiant’s Series A raise less than a year ago. Its contributions in the Series A raise helped Syntiant successfully execute its growth plan for 2018, and the rapid advancement of the company has significantly increased the value of Sunstone’s initial and continuing investment. Sunstone Management, with its subsidiary Sunstone Venture Capital Fund, is a diversified private capital management and investment firm offering comprehensive wealth management solutions to high net worth clients worldwide, in particular U.S. new-immigrant families.
“Syntiant’s innovations will bring tremendous value to the AI era. Our fund believes that this is one of the greatest venture opportunities on the market,” stated John Shen, founding partner of Sunstone Management. “We’re thrilled at their achievement with Series B and excited that this will enable us to continue to deliver returns to partners and investors in our Sunstone Venture Capital Fund.”
“We are both humbled and excited to be supported by several of the world’s greatest technology companies,” said Kurt Busch, CEO of Syntiant. “With participation from existing and new investors, and deeper guidance from our expanded board with the additions of Samir Kumar and Bret Johnsen, we will be able to commercialize Syntiant’s neural network technology for battery-powered devices to truly enable pervasive artificial intelligence.”
Tuff Yen, a senior fund manager of early round investments for startups and founder of Seraph Group, also both a Series A and B investor, added: “Syntiant has incredible business value due to its solutions to the high-power costs of moving and processing data to support advanced machine learning applications at the device level.”
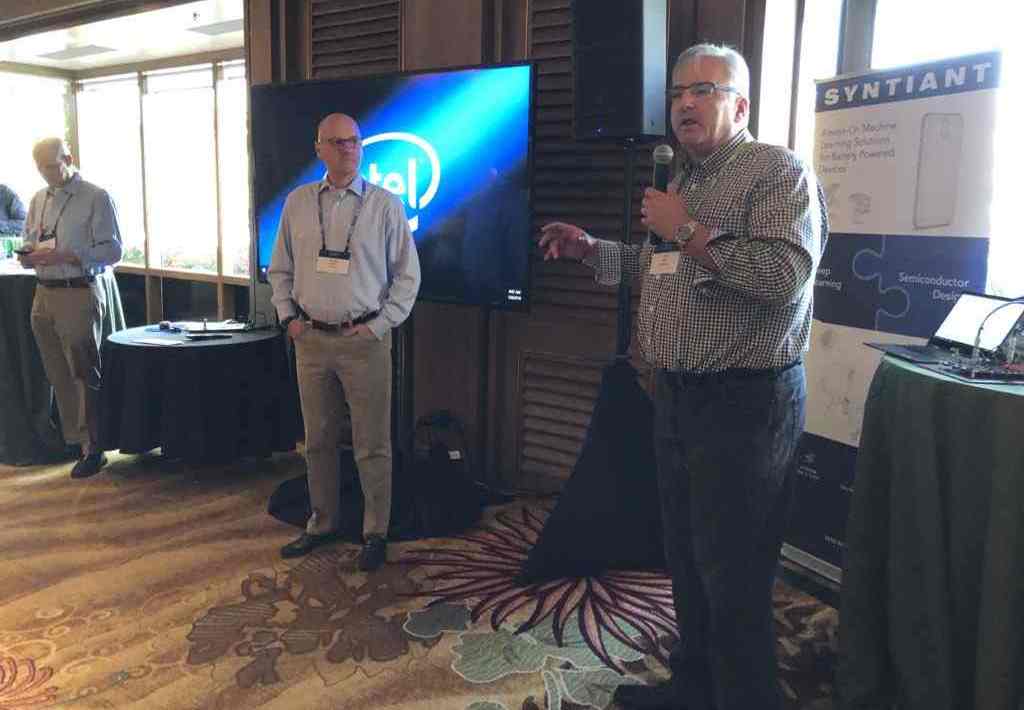
Syntiant CEO Kurt Busch addresses media prior to demonstrating Syntiant’s analog neural network technology at intelcapital’s ICAPSummit.