A new AI breakthrough developed by Microsoft will remove language barriers and help people around the world communicate better
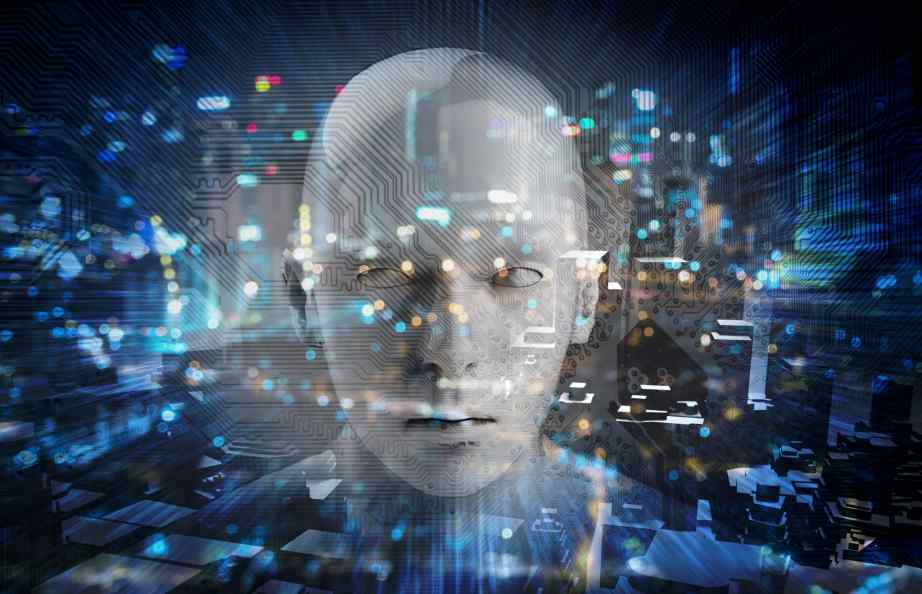
Since the Tower of Babel, humans have never been closer than it is today. With the global emergence of the Internet and advances in translation and speech technologies, it’s now possible to communicate with people around the world than any other time in history. Microsoft is about to accelerate the process even further with the creation of the world’s first the first machine translation system. Researchers at Microsoft’s Asia and U.S. labs said that they have developed a translation system that can translate sentences of news articles from Chinese to English with the same quality and accuracy as a person.
Machine translation has made substantial advances in recent years, thanks in part to advances in AI chip sets, more research & development, and better algorithms. Millions of people are using machine translation today in online translation systems and mobile applications in order to communicate across language barriers. The challenge has always been whether the approach achieve parity with human translation. So, the question then becomes, what is human parity? Microsoft provides two definitions in its 25-page research paper that defines human parity as: “If a bilingual human judges the quality of a candidate translation produced by a human to be equivalent to one produced by a machine, then the machine has achieved human parity.” Another definition is: “If there is no statistically significant difference between human quality scores for a test set of candidate translations from a machine translation system and the scores for the corresponding human translations then the machine has achieved human parity.”
In the blog post, the researchers claimed their system achieved human parity on a commonly used test set of news stories, called newstest2017, which was developed by a group of industry and academic partners and released at a research conference called WMT17 last fall. The team also hired external bilingual human evaluators, who compared Microsoft’s results to two independently produced human reference translations, to ensure the results were both accurate and on par with what people would have done.
Xuedong Huang, the leader of a group that recently achieved human parity in a conversational speech recognition task, called it a major milestone in one of the most challenging natural language processing tasks. “Hitting human parity in a machine translation task is a dream that all of us have had,” Huang said. “We just didn’t realize we’d be able to hit it so soon.”
Huang, a technical fellow in charge of Microsoft’s speech natural language and machine translation efforts, said the translation milestone was especially gratifying because of the possibilities it has for helping people understand each other better. “The pursuit of removing language barriers to help people communicate better is fantastic,” he said. “It’s very, very rewarding.” Researchers have worked on machine translation is a problem for decades – and, experts say, for much of that time many believed human parity could never be achieved. Still, the researchers cautioned that the milestone does not mean that machine translation is a solved problem.
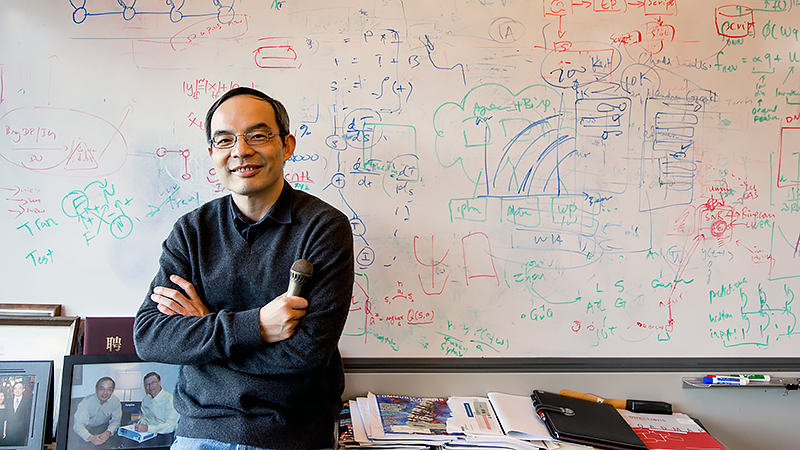
Story on Voice Recognition at Microsoft of Fil & XD on November 24, 2015. (Photography by Scott Eklund/Red Box Pictures)
This not the first time academic and industry researchers have worked on translation for years. However, with advances in AI chip set and better algorithms, they are now able to achieve greater breakthroughs by using a method of training AI systems called deep neural networks. That has allowed them to create more fluent, natural-sounding translations that take into account an even broader context than the previous approach, known as statistical machine translation.
To reach the human parity milestone on this dataset, three research teams in Microsoft’s Beijing and Redmond, Washington, research labs worked together to add a number of other training methods that would make the system more fluent and accurate. In many cases, these new methods mimic how people improve their own work iteratively, by going over it again and again until they get it right.
“Much of our research is really inspired by how we humans do things,” said Tie-Yan Liu, a principal research manager with Microsoft Research Asia in Beijing, who leads a machine learning team that worked on this project.
One method they used is dual learning. Think of this as a way of fact-checking the system’s work: Every time they sent a sentence through the system to be translated from Chinese to English, the research team also translated it back from English to Chinese.
Another method they used is called deliberation networks, is similar to how people edit and revise their own writing by going through it again and again. The researchers taught the system to repeat the process of translating the same sentence over and over, gradually refining and improving the response.
They also used one technique, called joint training, to iteratively boost the English-to-Chinese and Chinese-to-English translation systems. With this method, the English-to-Chinese translation system translates new English sentences into Chinese in order to obtain new sentence pairs.