Google AI Beats Doctors at Breast Cancer Detection—Spots Breast Cancer Missed by Human Eyes
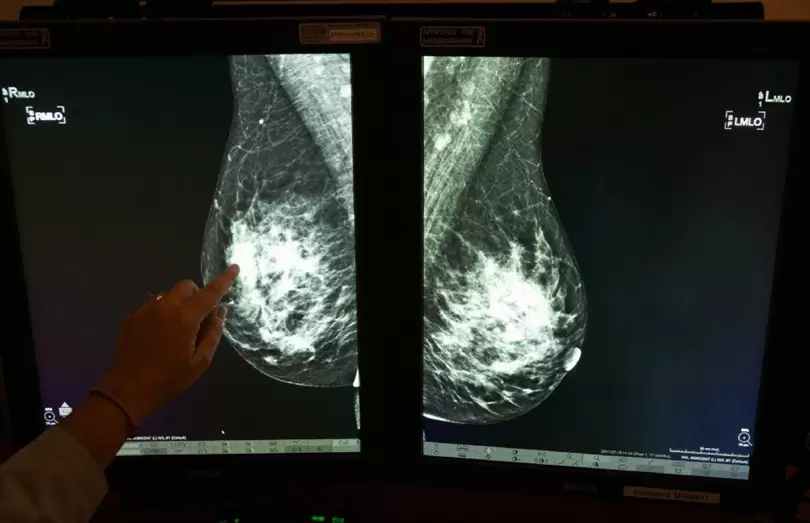
About one in 8 U.S. women (about 12%) will develop invasive breast cancer over the course of her lifetime. In 2019, an estimated 268,600 new cases of invasive breast cancer are expected to be diagnosed in women in the U.S., along with 62,930 new cases of non-invasive breast cancer, according to data from BreastCancer.org. That’s why early diagnosis and early detection is critical to preventing and treating breast cancer. But also important is the accuracy of the diagnosis.
Unfortunately the current and primary method of detection via mammogram, the process of using low-energy X-rays to examine the human breast for diagnosis and screening which is later examined by a radiologist, can result in a false diagnosis of cancer for many women. For others, it misses the cancer altogether. That’s why today’s Google’s Health AI discovery is a welcome news to everyone.
Today, DeepMind, a UK-based artificial intelligence startup purchased by Google in 2014, said it has developed an artificial-intelligence system that can match or outperform radiologists at detecting breast cancer. In some cases, however, doctors still beat the machines. Before now, DeepMind reported it developed similar AI systems for lung cancer, eye disease and kidney injury.
In collaboration with colleagues at DeepMind, Cancer Research UK Imperial Centre, Northwestern University and Royal Surrey County Hospital, the developed an AI model which can identify breast cancer from scans with fewer false positives or false negatives (when cancer is present but isn’t detected) than experts. Their model caught cancers that were originally missed and reduced false-positive cancer flags for patients who didn’t actually have cancer, according to a post published today In a post published by Shravya Shetty, M.S., Technical Lead, Google Health and Dr. Daniel Tse, Product Manager, Google Health.
The model was trained and tuned on a representative data set comprised of de-identified mammograms from more than 76,000 women in the U.K. and more than 15,000 women in the U.S., to see if it could learn to spot signs of breast cancer in the scans. The model was then evaluated on a separate de-identified data set of more than 25,000 women in the U.K. and over 3,000 women in the U.S. In this evaluation, our system produced a 5.7 percent reduction of false positives in the U.S, and a 1.2 percent reduction in the U.K. It produced a 9.4 percent reduction in false negatives in the U.S., and a 2.7 percent reduction in the U.K.
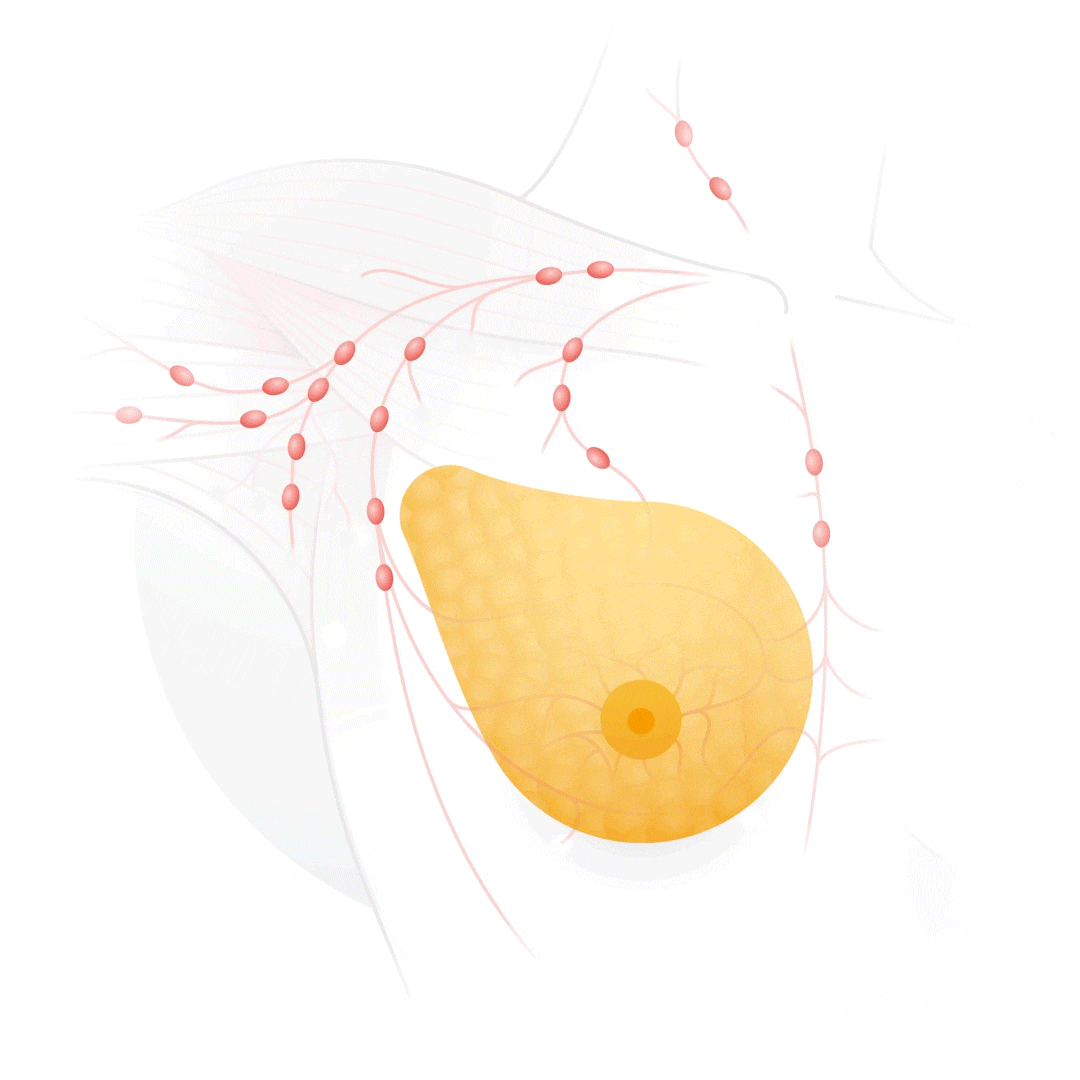
This is a visualization of tumor growth and metastatic spread in breast cancer. Screening aims to detect breast cancer early, before symptoms develop.
“This is another step along the way of trying to answer some of the questions that will be critical for us to actually deploying this in the real world,” says Dominic King, director and UK lead of Google Health. “This is another step closer to trying to deploy this type of technology safely and effectively.”
The system was first trained on de-identified mammograms from 76,000 British women, using Cancer Research UK’s OPTIMAM dataset, as well as 15,000 scans from the US. Once trained, the algorithm was tested on 25,000 scans in the UK, and a further 3,000 in the US. Four images from each mammogram were pulled into a neural network, spitting out scores for three different models between zero and one, with the latter a high risk of cancer.
The team also wanted to see if the model could generalize to other healthcare systems. To do this, they trained the model only on the data from the women in the U.K. and then evaluated it on the data set from women in the U.S. In this separate experiment, there was a 3.5 percent reduction in false positives and an 8.1 percent reduction in false negatives, showing the model’s potential to generalize to new clinical settings while still performing at a higher level than experts.
In November 2017, DeepMind announced a research partnership with the Cancer Research UK Center at Imperial College London with the goal of improving breast cancer detection by applying machine learning to mammography. Additionally, in February 2018, DeepMind announced it was working with the U.S. Department of Veterans Affairs in an attempt to use machine learning to predict the onset of acute kidney injury in patients, and also more broadly the general deterioration of patients during a hospital stay so that doctors and nurses can more quickly treat patients in need.