Perplexity AI’s claim that Sonar outperforms GPT-4o-mini and Claude 3.5 is misleading—Here’s why
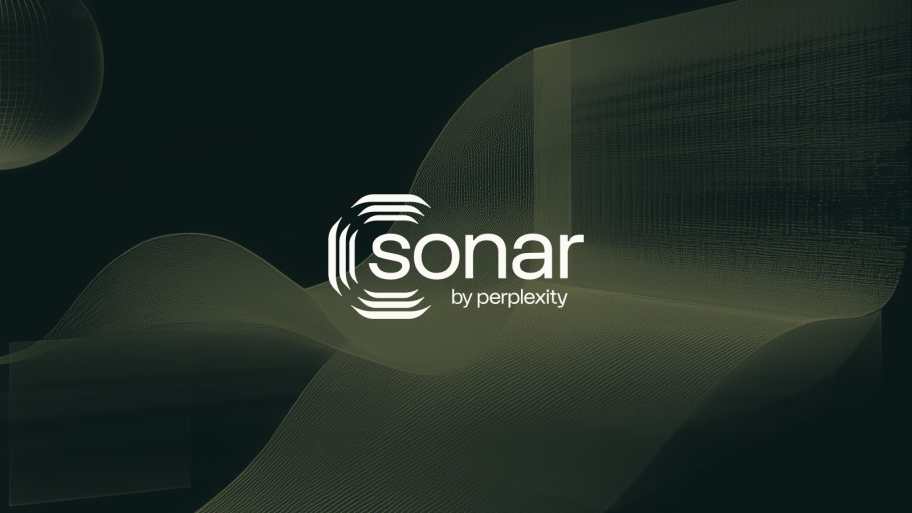
Perplexity AI is an AI search engine startup we featured here since its inception in 2022. In January, Perplexity announced the launch of Sonar, an advanced AI-powered search model built on Meta’s Llama 3.3 70B framework. It’s designed to deliver fast, accurate answers, optimized specifically for Perplexity’s search platform. They’re also touting Sonar‘s speed—processing up to 1,200 tokens per second—and its ability to provide high-quality, real-time responses using trusted web sources.
Then on Tuesday, Perplexity took to social media to hype up Sonar’s performance. In a post on X, Perplexity claimed that Sonar “outperforms GPT-4o-mini and Claude 3.5 Haiku while matching or surpassing top models like GPT-4o and Claude 3.5 Sonnet in user satisfaction.” Now, that might sound impressive on the surface, but the claim doesn’t tell the full story. In fact, it feels a little misleading without more context.
We get it—startups like Perplexity are always trying to push boundaries. But bold claims like this need to stand up to scrutiny, and this one raises a few questions.
Looking Closer at Perplexity’s Sonar Performance Claims
At face value, Perplexity’s statement suggests that Sonar isn’t just fast, but better than some of the most advanced models available—including OpenAI’s GPT-4o and Anthropic’s Claude 3.5 Sonnet. But “user satisfaction” is a pretty vague metric. Are they measuring speed, accuracy, the quality of responses, or something else? Without more details, it’s hard to know what they mean by “outperforms.”
And comparing Sonar to GPT-4o-mini and Claude 3.5 Haiku feels like stacking the deck. Both of those models are “lighter” versions of their more powerful counterparts, optimized for efficiency over peak performance. So, yeah, Sonar might outperform them—but that’s not exactly a fair fight.
Perplexity’s Sonar—built on Llama 3.3 70b—outperforms GPT-4o-mini and Claude 3.5 Haiku while matching or surpassing top models like GPT-4o and Claude 3.5 Sonnet in user satisfaction.
At 1200 tokens/second, Sonar is optimized for answer quality and speed. pic.twitter.com/cNhb39PEVV
— Perplexity (@perplexity_ai) February 11, 2025
Comparing a Porsche to a Truck
A better way to look at this is through a simple analogy: Large language models (LLMs) like GPT-4o and Claude 3.5 are like heavy-duty trucks 🚛—they’re built to handle massive workloads, from creative writing to coding and advanced reasoning. They carry a lot but move at a steady pace. On the other hand, Sonar is more like a Porsche 🏎️—lightweight, fast, and optimized for a specific purpose, in this case, retrieving real-time web data quickly.
Perplexity’s claim that Sonar is “faster” than models like GPT-4o is like saying, “Look, this Porsche is faster than a truck!” Sure, it is. But the truck wasn’t built for speed—it was built to haul a lot more weight. Comparing the two without mentioning their different purposes is misleading.
Another way to think about it is comparing a microwave to a chef. A microwave heats up food fast, but it doesn’t mean it’s better than a trained chef who can create a gourmet dish from scratch. Likewise, Sonar might retrieve facts faster, but that doesn’t mean it can think, reason, or create content on the same level as GPT-4o or Claude 3.5.
Speed Isn’t Everything
Perplexity claims Sonar can process 1,200 tokens per second, which is fast. But speed alone doesn’t make a model better. Quick responses are great, but if they sacrifice depth, coherence, or accuracy, what’s the point? Without solid benchmarks to prove Sonar maintains high-quality outputs at that speed, it just sounds like marketing fluff.
Why Transparency Matters
Perplexity AI’s claims about Sonar might sound impressive, but they lack the transparency needed to fully back them up. If “user satisfaction” is their key metric, we need to know how they’re measuring it and if it applies across different tasks—not just fact-based queries.
In an industry where accuracy and trust matter, bold statements without solid evidence can backfire. Sonar might shine in certain areas, but it’s still unclear if it really “outperforms” the biggest names in the LLM space.
Final Thoughts
Competition in AI is good—it drives progress. But companies need to be upfront about what their models can actually do. If Perplexity wants to compete with the likes of OpenAI and Anthropic, clearer benchmarks and more honest claims would help a lot. Until then, it’s up to users to dig a little deeper and see through the hype.